Why proactive data quality is essential for modern enterprises
Max Lukichev, CTO of Telmai, discusses why proactive data quality is essential, highlighting the pitfalls of traditional observability and how Telmai tackles data challenges head-on.
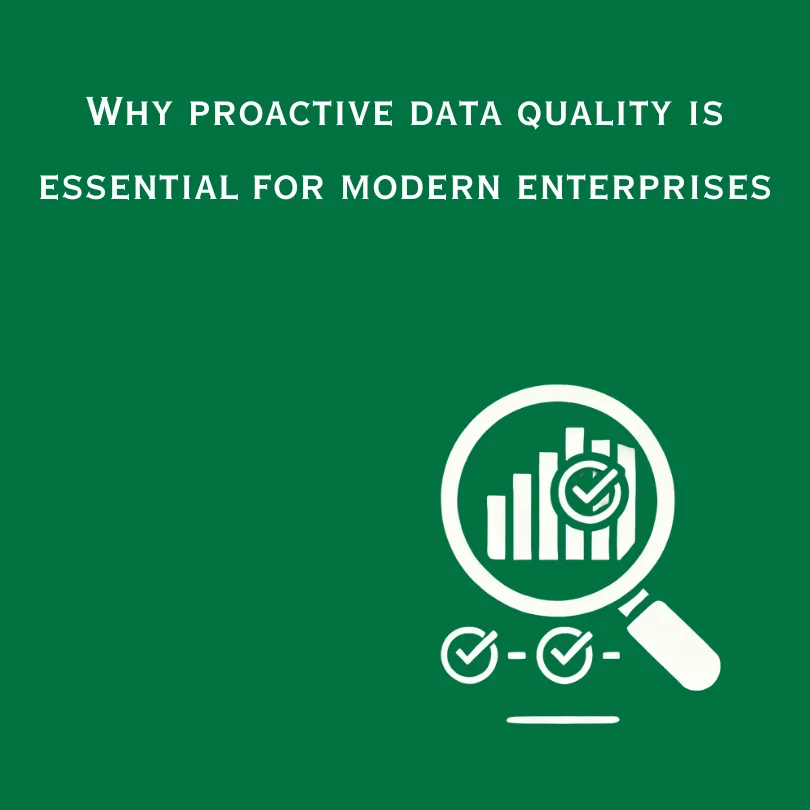
Data quality is a critical factor in the success of any organization, especially in the context of artificial intelligence and machine learning. From pipeline monitoring to AI-driven checks, the field of data observability has evolved to address the complexities of modern data ecosystems.
In an episode of the Behind Company Lines podcast, host Julian Torres spoke with Max Lukichev, co-founder and CTO of Telmai, about the evolution of data observability and the far-reaching impact of bad data on business outcomes.
Max Lukichev shares his journey from the early days of his career in established tech companies driven by a passion for solving data challenges head-on. Reflecting on his experiences at companies like Viva Systems and SignalFX, Max shared, “I’ve always been passionate about data, finding problems in data.” This passion led him to co-found Telmai, aiming to bridge gaps he saw in traditional data observability.
Shortcomings of traditional data observability
For many companies, data observability begins and ends with monitoring pipeline performance—ensuring data arrives on time, checking for errors in transmission, and verifying pipeline flow. While these are essential, they only scratch the surface of what’s needed to ensure reliable data.
Max points out that this approach leaves a gap in understanding the integrity of the data itself. “Most observability tools focus on pipeline flow, but the real value is in monitoring the data’s quality,” he explains. When data quality issues go unnoticed, they can lead to unreliable insights, poor decision-making, and significant operational setbacks.
The prevalence of “Garbage” data
Max highlighted the shocking reality that companies often have dozens to hundreds of data sources, both internal and external, and this data is rarely clean. “There’s so much garbage in the data, it’s just unbelievable,” Max stated. “And the most unbelievable thing is that people think they don’t have that problem until they actually see it.”
When unchecked, this low-quality, ‘garbage’ data skews analytics and compromises decision-making. Max points out, “All analytics is based on the assumption that data is of high quality. If you cannot ensure quality, it’s a waste of effort.” The problem only compounds once flawed data moves downstream, making remediation more complex, time-consuming, and costly. This reality underscores the need for proactive monitoring and quality control at the source to prevent costly setbacks and ensure data reliability.
A shift towards proactive data quality
Max advocates for a shift towards proactive data quality as part of comprehensive data observability. By monitoring the structure, format, and distribution of data, teams can catch irregularities before they escalate. “With a proactive approach, we’re not waiting for business users to spot inconsistencies. Instead, tools like Telmai help users identify and resolve these issues early on,” he shares.
This proactive stance helps companies avoid the typical delays associated with escalations or manual quality checks, streamlining the path from data generation to decision-making. For large enterprises, where data flows between numerous systems and departments, this can mean the difference between actionable insights and wasted effort.
Challenges in implementing data quality for enterprises
Max highlights the unique challenges that large organizations face when tackling data quality and observability. Enterprise clients often require tailored solutions, customized integrations, and thorough security evaluations, which makes implementing data quality practices complex. “Enterprise observability isn’t one-size-fits-all. Each client has unique needs, and scaling observability across a large organization requires adaptability,” he explains.
For these companies, the value of data quality goes beyond accuracy—it’s about enabling cross-functional teams to trust and act on data without hesitation. In an enterprise setting, where decision-makers depend on timely, accurate data, this kind of reliability is paramount.
Telmai’s unique approach to data quality
Telmai addresses these data quality and observability challenges by taking a proactive, data-centric approach. As Max explains, “We’re focused on catching issues as close to ingestion as possible,” meaning Telmai monitors not just pipeline flow but the data’s integrity right from the start.
Leveraging AI and machine learning, Telmai establishes a baseline for how data should look, identifying unusual patterns or errors before they become business disruptions. “We’re trying to remove that escalation path and be more proactive in fighting those issues,” Max shares, underscoring Telmai’s commitment to helping enterprises act on accurate, reliable data. This approach allows teams to avoid costly setbacks, creating a clear path to dependable, data-driven insights and empowering enterprises to operate on high-quality, reliable data.
Curious to learn more about Max’s journey, his approach to data quality, and his thoughts on the future of data observability?
Click here to access the full interview on Behind Company Lines with Julian Torres, where he shares valuable insights on transforming data management for the modern enterprise.
Passionate about data quality? Get expert insights and guides delivered straight to your inbox – click here to subscribe to our newsletter now.
- On this page
See what’s possible with Telmai
Request a demo to see the full power of Telmai’s data observability tool for yourself.