Unpacking data quality in the age of AI
Data quality is the backbone of AI success. In this blog, we explore how enterprise leaders address the foundational challenges of data quality in AI, and why ensuring reliability at every stage is essential for driving impactful business decisions.
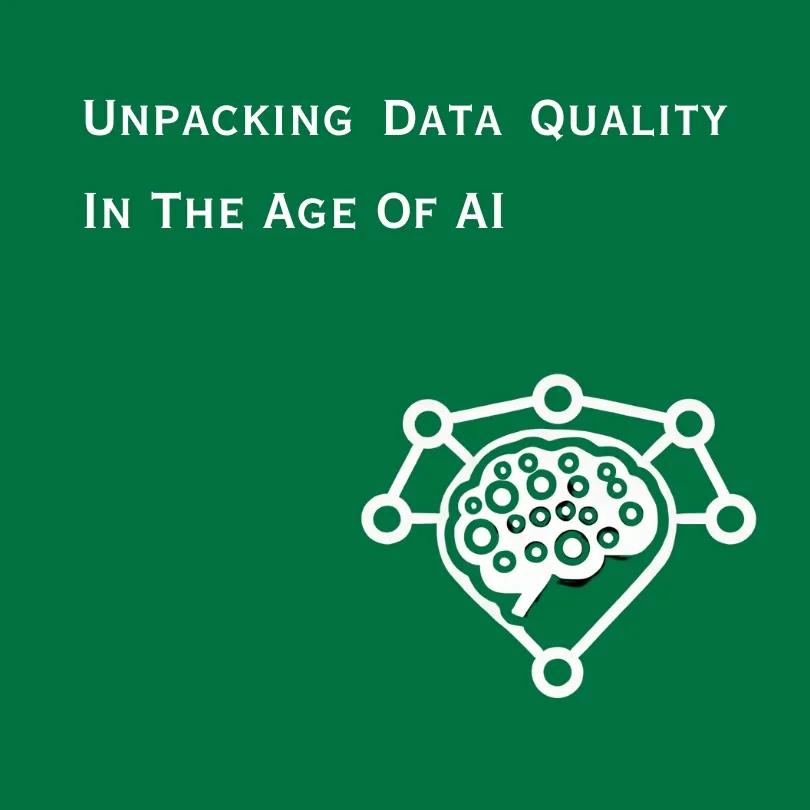
Data has become the lifeblood of decision-making and operational efficiency. However, as AI systems grow more powerful, they are only as good as the data they are built upon. This was at the heart of a recent panel discussion hosted by Ravit Jain and Mona Rakibe, where enterprise leaders like Ellen Nielsen, Appal Badi, and Miguel Navarro dove deep into the critical importance of data quality in productionisation of AI.
In “Unpacking Data Quality in the Age of AI,” the first episode of our new series focused on data quality; we explore how enterprises can manage data complexity, ensure reliable data pipelines, and create a culture of data accountability.
Data quality and business success
Good data powers good business decisions, while bad data leads to catastrophic results. Appal Badi chimed in on this by explaining that “data reliability and data quality is like forming the foundation of trust in terms of accuracy or consistency for data-driven decisions.” In sectors like finance, where regulations and compliance are paramount, ensuring data reliability is not just a technical concern but one that safeguards the entire decision-making process. When businesses rely on poor data, the risk of operational or regulatory failure becomes much higher, ultimately leading to a loss of trust both internally and externally.
Miguel Navarro added a more customer-facing perspective: “If you told them they’d have to wait seven days, they’d leave your organization.” His point stresses that clients now expect real-time access to accurate data, especially in domains like banking, where decisions and responses are expected to be instant. Inaccurate or delayed data not only frustrates clients but can result in losing them to competitors offering better, faster services.
Ellen Nielsen brought the conversation back to the fundamental impact on business operations, noting that “if you don’t have trust in your data, you struggle, and that immediately impacts business success.” Trust in data underpins nearly every business function, from marketing strategies to supply chain management. Without it, companies face inefficiencies and potentially costly mistakes.
The rising challenge of managing data complexity in productionizing AI
As AI becomes a significant part of business operations, the complexity of managing data increases dramatically. Companies now deal with massive volumes of data coming from a variety of sources—structured, unstructured, real-time, and historical. Ensuring the accuracy, consistency, and relevance of all this data is one of the most significant challenges for scaling AI implementations. With inaccurate or inconsistent data, AI models risk producing poor results or overfitting to flawed patterns. How Telmai can help with data quality? It offers advanced solutions for monitoring, cleaning, and integrating data to address these challenges, allowing businesses to manage data complexity with greater confidence.
Ellen pointed out that traditional methods of managing data are no longer sufficient: “The data is too unstructured and too complex for manual fixes.” In today’s world, where data is more unstructured than ever, companies can’t rely on manual data cleaning processes. Instead, they need advanced tools and techniques to automate the cleaning, transformation, and integration of data, especially when dealing with AI systems.
Miguel discussed how data complexity has increased as businesses pull information from multiple sources—“Today’s data is extremely complex because it spans multiple sources—mobile, desktop, on-prem, and cloud solutions.” This means that managing real-time data flows requires robust infrastructure to ensure that data remains consistent and accurate across all platforms. Without these systems, AI models can easily fall into traps of incorrect or incomplete data.
Appal noted that a cultural transformation is necessary to tackle this challenge: “The cultural transformation is required; data quality needs to happen at the data entry or process integration point.” Rather than waiting for errors to snowball downstream, companies need to embed data quality checks at the point of data entry. This proactive approach helps to prevent bad data from entering the system and ensures that AI models are trained on reliable data from the start.
Cultural and organizational shifts in ensuring data quality
Data quality isn’t just a technical issue—it’s a cultural one. In many organizations, data management has been siloed, with the responsibility for quality resting solely on IT or specialized teams. But in the AI age, everyone in the organization—from the data producer to the consumer—must play a role in maintaining data quality.
Appal emphasized this need for shared responsibility: “Accountability for data quality has to be federated—it should not sit solely with the central team but across all parts of the organization.” In his view, data quality should not be the burden of one department. Every team that interacts with data must take ownership of the quality of that data, ensuring consistency and accuracy from end to end.
Ellen added that while processes and technologies are essential, the real change begins with people: “Don’t start with the process and technology—start with your people.” Ellen’s perspective is that the human element is often overlooked when tackling data quality issues. While tools and systems can certainly help, the employees who ultimately interact with and produce data need to be aligned on quality goals.
Miguel likened data quality to a continuous, team-wide effort: “Data quality is a team sport. It’s always continuous; there is no on and off switch.” For Miguel, maintaining data quality is not a one-off task—it requires ongoing vigilance and collaboration across departments to ensure that data remains accurate and reliable as it flows through the organization.
The role of leadership in data quality initiatives
Leadership plays a crucial role in driving data quality initiatives. Without the support and vision of senior leaders, efforts to improve data quality often stall or become fragmented. Leaders must not only advocate for high-quality data but also provide the resources and cultural support necessary to embed data quality into the fabric of the organization.
Ellen also stressed that leadership needs to start small to gain traction: “You cannot always boil the ocean; you need to start with small, manageable cases to gain leadership support.” Rather than attempting to solve all data quality issues at once, leaders should focus on small, high-impact projects that demonstrate the value of data quality and build momentum for broader initiatives.
Miguel suggested a balanced approach: “It needs to be a sandwich approach: leaders empower people, and people on the ground create trust and collaboration.” Leaders provide the necessary vision and support, while those on the ground implement the processes that ensure data quality at every stage. This collaboration between leadership and teams makes data quality initiatives sustainable.
Appal highlighted the importance of leadership’s role: “Data quality should be embedded by design—it’s a cultural transformation that must start at the top.” He emphasized that without leadership driving the importance of data quality, it’s difficult for teams on the ground to make meaningful progress.
Data observability and AI: an evolving landscape
As AI becomes more integrated into business processes, data observability tools are emerging as critical components for ensuring data quality. These tools allow businesses to monitor their data pipelines in real-time, checking for anomalies, data drift, and other quality issues that can impact AI models.
Appal Badi explained how these tools are transforming the landscape: “Data observability tools are bringing together the key pillars of schema, freshness, volume, and quality in a unified approach.” By providing visibility into every aspect of data quality, observability tools help ensure that AI models are fed accurate, up-to-date data, resulting in more reliable outputs.
Ellen Nielsen used a thoughtful analogy to explain the importance of patience in improving data quality: “You cannot rush data quality—like fine wine, it matures over time.” Building robust data quality systems is a long-term investment. Rushing the process often leads to failures, whereas a careful, measured approach yields better results in the long run.
Miguel Navarro added,” The delivery and velocity of data are critical to AI success, and companies must figure out how to manage data observability while ensuring security.” With real-time data pipelines, businesses must balance speed and security, ensuring data flows quickly without compromising quality or exposing sensitive information.
Conclusion
Data quality is the cornerstone of any successful AI initiative. As discussed in the panel, managing the complexity of modern data flows, fostering accountability across teams, and adopting the right tools for data observability are all crucial for ensuring that AI systems deliver reliable and valuable insights. Leadership plays a vital role in embedding data quality into the organization’s culture. With the proper support, enterprises can unlock the full potential of AI and data-driven initiatives.
Want to dive deeper into these insights? Click here to check out the full interview for a comprehensive discussion on how to tackle data quality challenges in productionising AI.
Passionate about data quality? Get expert insights and guides delivered straight to your inbox – click here to subscribe to our newsletter now.
- On this page
See what’s possible with Telmai
Request a demo to see the full power of Telmai’s data observability tool for yourself.