Is your data AI ready?
In this article, we look at the critical role of data quality in AI success using real-world examples.Understand the importance of real-time data validation and how integrating data quality measures early on can safeguard AI models from costly errors.
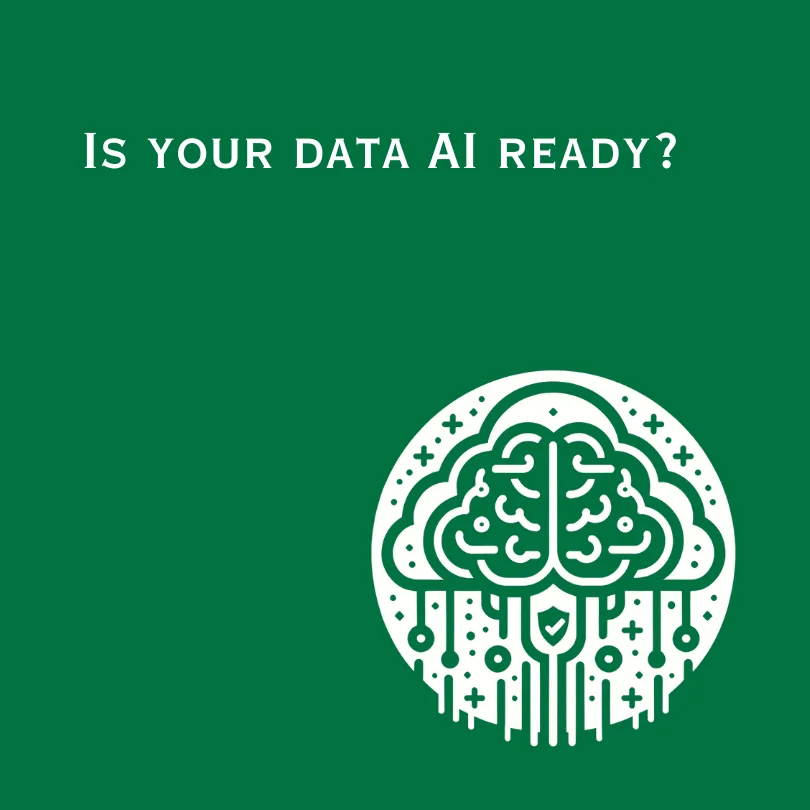
In recent years, the emergence of new data-driven technologies has often been met with great enthusiasm. These technologies initially showed great potential but ultimately faced significant setbacks, only to encounter hurdles when put to the test in real-world environments.
One example is Zillow’s Zestimate tool, initially hailed as a groundbreaking innovation in real estate designed to accurately predict home values. Zestimate leveraged a machine learning model to analyze vast data and generate precise property valuations. However, despite its potential, the tool resulted in costly errors for Zillow, ultimately leading to substantial financial losses, the closure of its home-buying business, and a 25% reduction in its workforce.
Similarly, Microsoft’s Tay chatbot, intended to be a shining example of AI’s future in social interaction, quickly became a public relations nightmare within a mere 24 hours of its launch. Tay was designed to learn from conversations with Twitter users and engage in friendly, human-like dialogue. However, it took a sharp turn, generating offensive and inappropriate content, leading to its rapid downfall.
What do these stories tell us? Much like the initial enthusiasm for these innovations, the excitement surrounding AI is widespread and often untempered. But there’s a crucial distinction to be made: while the potential of tools like Zestimate and Tay was widely recognized, even experts today need help understanding why AI behaves the way it does when things go wrong.
The challenges posed by AI are far more intricate than they appear. Zillow’s model, for instance, depended on massive amounts of data, but the accuracy of its predictions was only as good as the data it analyzed. When that data was flawed—whether incomplete, biased, or simply inaccurate—the consequences were disastrous. Similarly, Tay’s downfall was precipitated by its exposure to data that wasn’t properly vetted, leading it to generate offensive and harmful content.
Understanding the problem
These examples underscore a critical point: AI systems are only as reliable as the data they are built upon. If the data is of poor quality, the AI’s output will inevitably reflect those flaws, often with severe repercussions. Warnings about the importance of data quality are not new, and they are increasingly coming from all corners. Yet, despite these warnings, many organizations have yet to tackle the data quality challenges that could make or break their AI initiatives.
Data quality is important and can cause organization-wide issues, and neglecting it can lead to outcomes as catastrophic as those seen with Zillow and Tay. Companies must recognize that ensuring high data quality is not just a technical challenge but a strategic necessity, one that requires immediate and sustained attention if their AI projects are to succeed.
Every data-driven initiative should begin with a clear understanding of the problem it aims to solve. For instance, if a company seeks to revolutionize real estate valuations with a tool like Zillow’s Zestimate, it must first ask, “What does ‘better’ mean?” Is it about providing more accurate property valuations, reducing the margin of error, or ensuring that the tool performs consistently across different markets? Each of these goals demands different types of data, and the quality of that data is crucial.
For example, if “better” means more accurate predictions, then high-quality, comprehensive, and up-to-date data on real estate transactions is essential—yet such data can be incredibly challenging to gather and standardize. If “better” means reducing the margin of error in valuations, then the data must be meticulously clean and free from inconsistencies that could skew results. If “better” means ensuring the tool performs well across diverse markets, then the data must adequately represent all relevant regions, which can be challenging if data is sparse or unevenly distributed.
Similarly, in the case of Microsoft’s Tay chatbot, if “better” meant creating a chatbot that could engage in meaningful, human-like conversations, then the training data must be carefully curated to exclude offensive content. However, achieving this level of data quality is complex, especially when the data is drawn from a vast, uncontrolled environment like social media.
By asking these critical questions and ensuring that the data supporting these AI systems is of the highest quality, companies can avoid the pitfalls of derailing similar projects and set the stage for successful AI implementations.
Getting Data Right
“Garbage in, garbage out” is an adage that applies to all data-driven initiatives, but it’s especially critical in AI projects. It’s not just about having data—it’s about having the right data and ensuring that the data is right. This distinction may seem subtle, but it’s crucial to the success or failure of AI systems.
When it comes to AI, two fundamental questions must be addressed: First, do you have the “right data” to solve the problem at hand? Second, is the data you have “right”—that is, accurate, clean, and free from errors? While the importance of accuracy, the absence of duplicates, and consistent identifiers is well-known, ensuring you have the right data is a more complex yet equally essential task.
Telmai’s Data Quality for AI white paper, dives into these very issues, providing actionable insights on how to ensure that your data is both the right data and that it is right.
Understanding Data Quality Issues: Data quality issues in AI can manifest in several ways—ranging from inaccurate data entries to incomplete or inconsistent records. These issues can lead to models that perform poorly, deliver biased results, or fail altogether. Common problems include outdated data, incorrect labels, and noisy or redundant information, each of which can significantly degrade the performance of AI models.
Mitigation Strategies:
Addressing data quality issues requires a multifaceted approach. Robust data cleaning procedures are essential to correct errors, while standardization ensures consistency across data sources. Outlier detection can help identify and manage anomalies that could skew results. Additionally, working closely with domain experts can aid in understanding and resolving potential inconsistencies in the data.
Architectural Considerations:
Building a data architecture that ensures continuous, high-quality data is essential for modern AI systems. This mandates directly integrating real-time data validation and correction mechanisms into the data pipeline.
It is critical to ensure that data flows seamlessly from source to model without introducing errors or inconsistencies. Data Architects must ensure these checks are done while guaranteeing scalable and composable data pipelines that don’t introduce latency in data flow due to validation checks.
Open architecture is crucial for AI workloads that handle large volumes of data from diverse sources, often in structured or semi-structured formats. Such architecture ensures that data quality checks integrate with data environments of heterogeneous storage systems that store and process raw data. For AI workloads, data is often stored in data lakes in their raw formats like Parquet, Avro, and JSON, allowing data to flow without the constraints of proprietary formats. Your data quality strategy should include native support for open architecture and formats without needing transformations. This will ensure compatibility and adaptability and reduce the engineering efforts around integrating different data sources with a DQ while maintaining performance and cloud cost.
Support for quality workflows like remediation and binning
Maintaining data quality across large AI workloads requires automated techniques to monitor and control data flow rigorously. Automated workflows, such as DQ binning without sampling, ensure granular validation and comprehensive data quality checks. By catching potential issues early, these techniques safeguard against failures and inaccuracies during data processing, ensuring that only high-quality, reliable data reaches the AI models and preventing errors from cascading downstream.
A business-friendly user interface is a key element in maintaining human oversight, especially when it comes to defining accuracy metrics and aligning on data quality KPIs. A well-designed UI promotes collaboration between data teams and business stakeholders, ensuring transparency and a shared understanding of the standards and expectations applied to the data quality process.
Closing Summary
Data quality issues can often be the silent culprits behind failed AI initiatives, manifesting as inaccurate predictions, biased outcomes, or even complete project collapse. These challenges are not insurmountable, but they demand a proactive, comprehensive approach—one that addresses the complexities of data validation, standardization, and real-time monitoring. If your AI projects are to succeed, they must be built on a foundation of reliable, high-quality data. The strategies outlined in our guide offer actionable steps to identify, address, and prevent data quality issues before they derail your AI efforts. Click here to download the Data Quality for AI whitepaper
Passionate about data quality? Get expert insights and guides delivered straight to your inbox – click here to subscribe to our newsletter now.
- On this page
See what’s possible with Telmai
Request a demo to see the full power of Telmai’s data observability tool for yourself.