How data observability drives success in modern data architectures
Data teams are discovering the importance of Data Observability and its distinction from traditional data quality checks. This article explores four reasons why data observability is crucial for modern data stacks, the unique benefits of Telmai, and how it addresses the complexities of today’s data ecosystems.
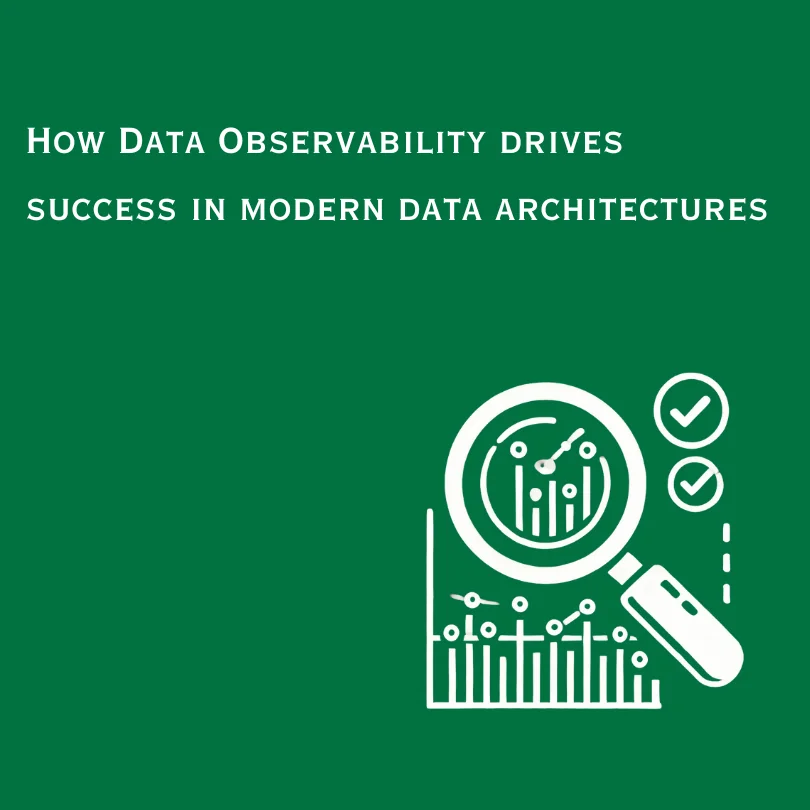
As data ecosystems grow increasingly complex, Traditional data quality checks are struggling to keep up and fall short, leaving data teams in search of more effective solutions. Data observability takes a proactive approach, going beyond traditional monitoring to predict and prevent data issues before they affect your business.
In a recent interview with Swapnil Bharatiya, CEO of TFiR, Mona Rakibe, co-founder and CEO of Telmai, shared her insights on why data observability is crucial for modern data stacks.
With growing investments in data and analytics, along with the explosion of new data products, companies now navigate a complex web of data pipelines. Data engineers are employed to manage these pipelines, troubleshoot issues, and prevent downstream problems. However, traditional data quality checks based on predefined rules have become too unpredictable and insufficient, necessitating a more advanced approach.
Key advantages of data observability in modern data stacks
1. Proactive detection of unknown issues using ML
In the past, data quality engines were set up through rules. However, rules-based systems don’t cut it anymore. Rules break as data changes over time. Mona Rakibe explains, “Rules-based systems don’t cut it anymore. The dynamic nature of data today demands a more advanced approach.” Instead of configuring and re-configuring what to check for, data observability relies on unsupervised learning and detects anomalies and outliers even if it was not programmed to do so. By using time series and historical analysis of data, data observability tools create a baseline for normal behavior in data and automate anomaly detection when data falls outside historical patterns or crosses certain thresholds. This proactive method ensures that even unanticipated issues are caught early, maintaining the integrity of your data.
2. Real-time monitoring and alerting
Data observability tools continuously monitor data flows and alert teams to anomalies or drifts. With this approach, you can automate quality checks and flag faulty data values as often as they happen and before any downstream impact. Mona highlights the importance of this feature, stating, “Real-time monitoring is a game-changer. By continuously monitoring data streams, we can catch and address issues instantly, often before they cause any significant damage.” You can set up monitors to run on an hourly, daily, or weekly basis and automatically see alerts and notifications via Slack or email when your data falls outside expected norms. For organizations using BigQuery, tools like BigQuery Data Observability can enhance these capabilities, providing real-time insights and flagging anomalies as soon as they arise.
3. Root cause investigation
When data quality issues are exposed, data observability tools have the means to show the root cause of these issues. The root cause analysis exposes the underlying data values and patterns contributing to faulty data. Mona elaborates, “Understanding the root cause is vital for effective resolution. Data observability tools provide deep insights into where and why issues occur, allowing teams to fix problems at the source quickly.” Data lineage further expands these discoveries to expose associated tables, columns, and timestamps of outliers, pattern changes, or drift in the data as soon as the change occurs. This helps data teams remediate these issues faster, significantly reducing downtime and enhancing overall data quality.
4. Shared data quality ownership
While traditionally, data quality management was done by IT because of the technical nature of tools, it was never clear who is the data quality owner. This led to much firefighting and dealing with data issues between teams. Mona addresses this issue, “One of the biggest challenges was the lack of clear ownership over data quality. Data observability bridges this gap by providing a user-friendly interface that both technical and business teams can use.” With data observability, a visual, no-code interface facilitates collaboration between business and technical teams. This intuitive interface helps them directly see data quality issues in motion, learn from historical trends, and establish validation rules to monitor data quality without having to code or go back and forth on business policies. This collaborative approach ensures everyone is on the same page, significantly reducing miscommunication and improving overall data governance.
What sets Telmai apart
While most data observability tools have some things in common, including the 4 we outlined above, they have been built with different architectures and for different use cases.
The right combo of ML and data validation rules
“Telmai learns from your data, identifies issues and anomalies as they occur, and predicts expected thresholds out of the box,” Mona explains. Telmai combines unsupervised learning with customizable rules and expectations, providing a powerful yet flexible solution. “This combination of unsupervised and supervised learning gives you the flexibility to tailor your data quality monitoring to your specific needs,” she adds.
Data quality, regardless of the data type
Traditionally, data quality was part of an ETL flow, with data being cleaned as it transformed into a reporting layer. “Today, data is sourced from various places, and transformations happen at different stages, whether in databases, source systems, or target systems,” Mona notes. With Telmai, data quality checks can be plugged in anywhere in your pipeline, regardless of the data type (structured, semi-structured, streaming) or storage (cloud warehouse, data lake, blob storage). “You are not limited to data sources with a SQL interface or strong metadata,” Mona emphasizes.
With Telmai, you can plug data quality checks anywhere in your pipeline, regardless of the data type (e.g. structured, semi-structured data, streaming) or data storage (e.g. cloud warehouse, data lake, blob storage) you have in place. You are not limited to data sources that have a SQL interface or carry strong metadata to help you infer data quality at an aggregated level.
Data quality at scale and volume
“Using Telmai, you can analyze the quality of your data at the attribute level in its full fidelity,” Mona explains. Telmai allows you to see data quality issues at any given point in time, as well as historically and continuously, for always-on monitoring. “You are not limited to samples that hide data quality issues or the number of data validation queries you can run. Telmai’s scalable Spark architecture ensures you won’t clog up the performance of your underlying data warehouses,” she highlights.With Telmai, You are not limited to samples that will hide data quality issues. You are also not limited to the amount of data validation queries you run against your database. Telmai analyzes data quality in its own scalable, Spark architecture so that you won’t clog up the performance of your underlying data warehouses.
Low TCO
“Built-in automation, ML-based anomaly detection, and out-of-the-box data quality metrics save you time and resources, both in setting up and maintaining your data validation processes,” Mona states. Telmai’s Spark-based data quality analysis layer eliminates the need to push validation rules and SQL queries into your analytic databases, reducing licensing costs. “With Telmai, you get powerful data quality monitoring without the high cost,” Mona assures.A future-proof architecture
As your data stack changes, you don’t have to change your data quality logic, SQL code, or native scripts that were previously investigating your data. With Telmai, you have an open architecture that can validate a field in Snowflake precisely the same as in Databricks or even a system with no SQL interface or a strong metadata layer – all within a no-code user-friendly interface.
Future proof your data stack
“As your data stack evolves, you won’t need to change your data quality logic, SQL code, or native scripts,” Mona explains. Telmai’s open architecture can validate a field in Snowflake just as effectively as in Databricks or a system without a SQL interface or strong metadata layer. “All of this is managed within a no-code, user-friendly interface,” Mona adds, emphasizing the ease of use and adaptability of Telmai’s solution.
Discover how Telmai can transform your approach to ensuring Data Quality. Try Telmai today!
Passionate about data quality? Get expert insights and guides delivered straight to your inbox – click here to subscribe to our newsletter now.
- On this page
See what’s possible with Telmai
Request a demo to see the full power of Telmai’s data observability tool for yourself.