Maximizing data systems architecture: A product-centric approach
Dive into Telmai’s journey of building a streaming analytics engine. Learn about Lambda and Kappa data architectures, and the tech stacks involved. A must-read for data systems enthusiasts.
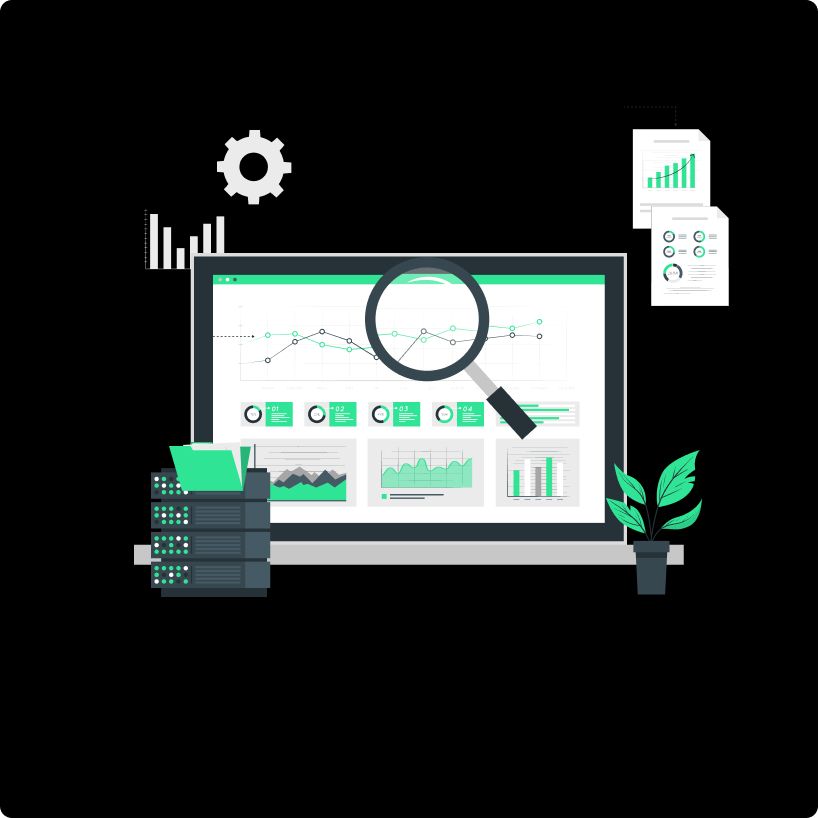
In a session hosted by Alexey Grigorev of DataTalks.Club featuring Maxim Lukichev, Co-Founder and Chief of Technology of Telmai, where he presented a revolutionary strategy for developing data systems. Max, whose expertise spans from query optimization to SaaS Data Management, advocated for a shift in perspective. He proposed treating data systems architecture as an integral part of an organization’s main product, moving beyond seeing it as just a technical challenge.
Transforming data systems architecture into a core product
“Adopting the product manager’s ethos,” Max advises, means shifting our view of data system architecture from just fixing problems to innovating and creating systems that truly meet user needs and adapt to changing market dynamics. He suggests a fundamental change in how we think about and work with data systems: “It’s about seeing beyond the immediate fix.”
Key strategies for a product-centric data architecture
Max outlines clear steps for making data systems architecture more product-centric:
1. Embrace Forward-Thinking Design
“Design your data quality solution to solve these problems first,” Max highlights, pointing out the importance of understanding the end-users’ needs and pain points. It’s not just about addressing current issues but also about planning for future growth and changes. This approach was key in his work at Reltio and SignalFx, focusing on “scalability and adaptability.”
2. Engineering for Excellence
Choosing the right technologies and architectures is critical. Max shares, “Our big data stack was Cassandra, Elasticsearch, and Spark… high scale and multi-tenancy.” This decision underpins the importance of building systems that can handle growing data volumes and complexity without faltering.
3. Prioritize Rigorous Testing
Testing is non-negotiable. Max emphasizes, “Testing is critical… not enough to assume your data quality initiatives are working; you must prove it through rigorous testing.” Setting up automated tests for various metrics ensures the system works as expected and remains reliable over time.
4. Ensure Seamless User Adoption
“User adoption is the ultimate test of your data quality strategy’s success,” He points out, stressing the need for clear training, documentation, and support. Making sure users are comfortable and proficient with the new system or updates is crucial for the system’s overall success.
5. Implementing KPIs for Continuous Improvement
“Define clear KPIs… these KPIs should align with your business objectives,” Max advises. It’s about measuring what matters most to your organization and using those metrics to drive ongoing improvements in your data system’s architecture.
Realizing the future of data architecture
Maxim Lukichev’s insights illuminate a transformative path for data systems architecture, advocating a shift from viewing it as merely foundational to recognizing it as a beacon of innovation. Embracing a “product manager’s ethos,” where he calls for a product-centric approach that aligns data infrastructures with the dynamic demands of the market. This vision emphasizes the necessity of creating systems that are not only scalable and adaptable but also maintain organizational agility in a fast-evolving technological landscape. Integrating this advanced methodology becomes crucial as entities seek to elevate their data systems into strategic, value-centric assets.
In this data-centric era, where strategic agility is paramount, Telmai stands out offering a solution that embodies the principles of a product-centric approach to data systems architecture. With its advanced machine learning-driven anomaly detection, Telmai ensures proactive identification and real-time monitoring of data quality KPIs. Also, Telmai enhances data operations with its left-shift-oriented, open architecture that integrates directly within the customer’s Google Cloud Platform (GCP) account. This approach optimizes performance by facilitating direct interaction with BigQuery and expands analytical capabilities without requiring data movement, ensuring peak performance without compromise.
Discover how Telmai can revolutionize your data ecosystem, making it more dynamic and value-driven. Request a demo of Telmai today.
Passionate about data quality? Get expert insights and guides delivered straight to your inbox – click here to subscribe to our newsletter now.
- On this page
See what’s possible with Telmai
Request a demo to see the full power of Telmai’s data observability tool for yourself.