Why modern data quality needs a new approach
In a recent chat, Nick Heudecker sat down with Mona Rakibe, the co-founder and CEO of Telmai, to explore the evolving world of data observability. Mona shared why traditional data quality methods are no longer enough and how modern approaches using machine learning and comprehensive pipeline monitoring can drastically improve data reliability.Discover key insights and practical tips for enhancing data quality in today’s dynamic business environment.
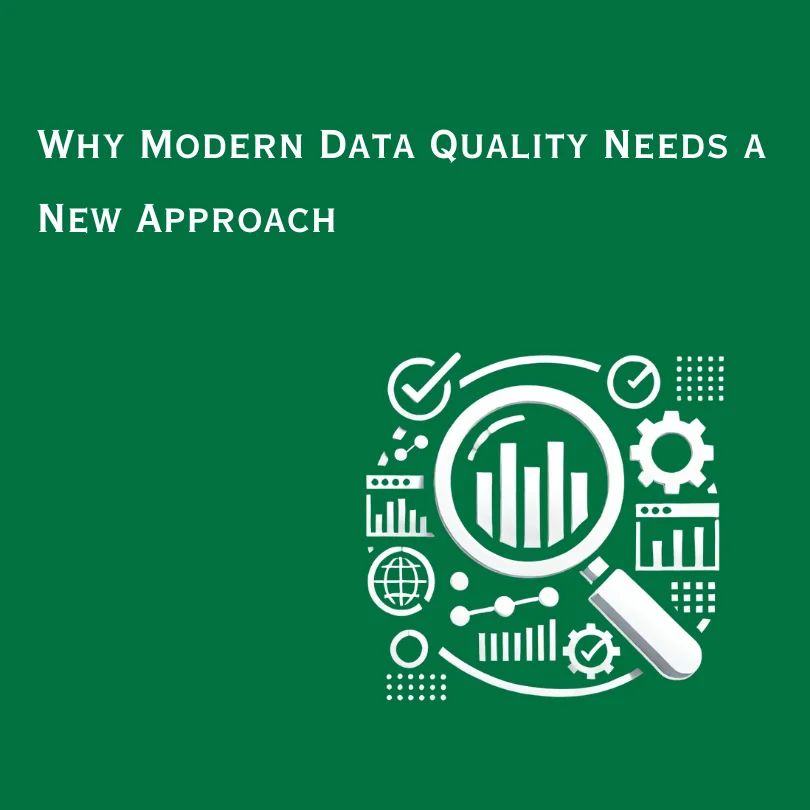
In a recent chat on the Data Mesh Learning Channel, Nick Heudecker sat down with Mona Rakibe, the co-founder and CEO of Telmai, to discuss the ever-changing world of data observability. Mona shared why this concept is so important for businesses and how it can drastically enhance data quality.
Here’s a rundown of their conversation and the main points that any data-driven organization should know.
The need for data observability & data quality methods
Data quality has always been important, but as Mona explained, the traditional methods of ensuring data quality are no longer sufficient. “Data quality existed from the time that data existed, but the volume, velocity, and variety of data today make traditional methods insufficient,” she said. Traditional data quality tools often rely on fixed rules and policies, which can’t keep up with the dynamic nature of modern data ecosystems. Mona pointed out, “Rules don’t scale for this type of architecture and this type of data ecosystem.” Instead, using statistical analysis and machine learning allows for a more flexible and adaptive approach to maintaining data quality.
The role of machine learning in data observability
One of the key innovations in data observability is the use of machine learning to automatically detect and address data issues. Mona emphasized this point, stating, “Simple thresholds like row count cannot stay consistent because your business is changing month over month.” Machine learning can take into account these changes and automatically adjust thresholds, making it easier to spot anomalies and maintain high data quality.
Mona also discussed the importance of monitoring the entire data pipeline. “We support multiple formats, JSON, CSV, Parquet, and multiple data sources like data lakes, S3, GCS, BigQuery, and Azure Blob,” she explained. By providing end-to-end monitoring, organizations can ensure that data remains reliable from its source to its final destination. Customization is also crucial because different teams within an organization may have different requirements. Mona highlighted, “In our world, N/A should be treated as null and should be a completeness metric, and that should be different for our financial ops team versus marketing.”
Addressing the growing complexity of data roles
As data becomes more integral to business operations, new roles are emerging to manage it. Mona expressed her excitement about this trend, saying, “I cannot tell you how excited I feel when I see a data quality product manager, data product managers, where there’s such a good focus on treating data as a product.” This shift reflects the increasing importance of data quality and the need for specialized roles to manage it effectively.
Looking ahead, Mona believes the field of data observability will continue to grow and evolve. “There’s a humongous potential for every company to adopt data observability and get to their goals of data reliability,” she said. As companies recognize the critical importance of data reliability, tools and practices in this space will continue to advance.
Final thoughts
Data observability is a crucial component of modern data management. By moving beyond traditional methods and embracing machine learning and comprehensive pipeline monitoring, businesses can ensure their data is reliable and valuable.
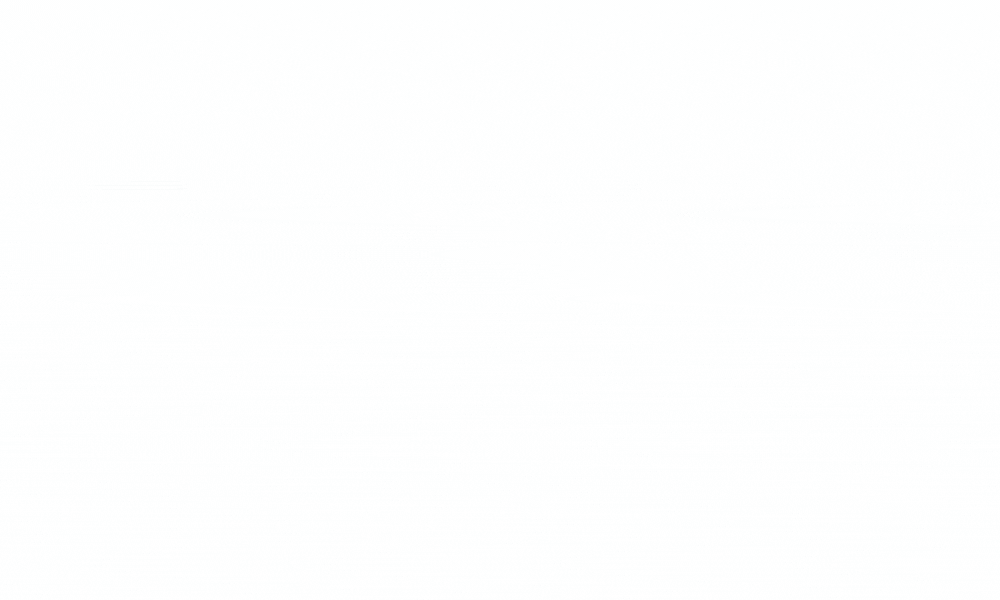
If your organization uses a modern data stack, you should use a modern data quality tool like Telmai.
Telmai stands out for its rapid deployment, ease of maintenance, and intelligent, always-on monitoring that delivers insights swiftly. As an AI-based data observability platform designed for open architecture, Telmai excels in real-time monitoring across the data pipeline, from data warehouses to cloud storage systems. It proactively detects anomalies in both structured and semi-structured data sources, ensuring decisions are based on reliable data and preventing bad data from impacting critical decisions.Discover how Telmai can revolutionize your data ecosystem. Request a demo today.
Passionate about data quality? Get expert insights and guides delivered straight to your inbox – click here to subscribe to our newsletter now.
- On this page
See what’s possible with Telmai
Request a demo to see the full power of Telmai’s data observability tool for yourself.